The Key Differences Between Data Analytics & Data Science
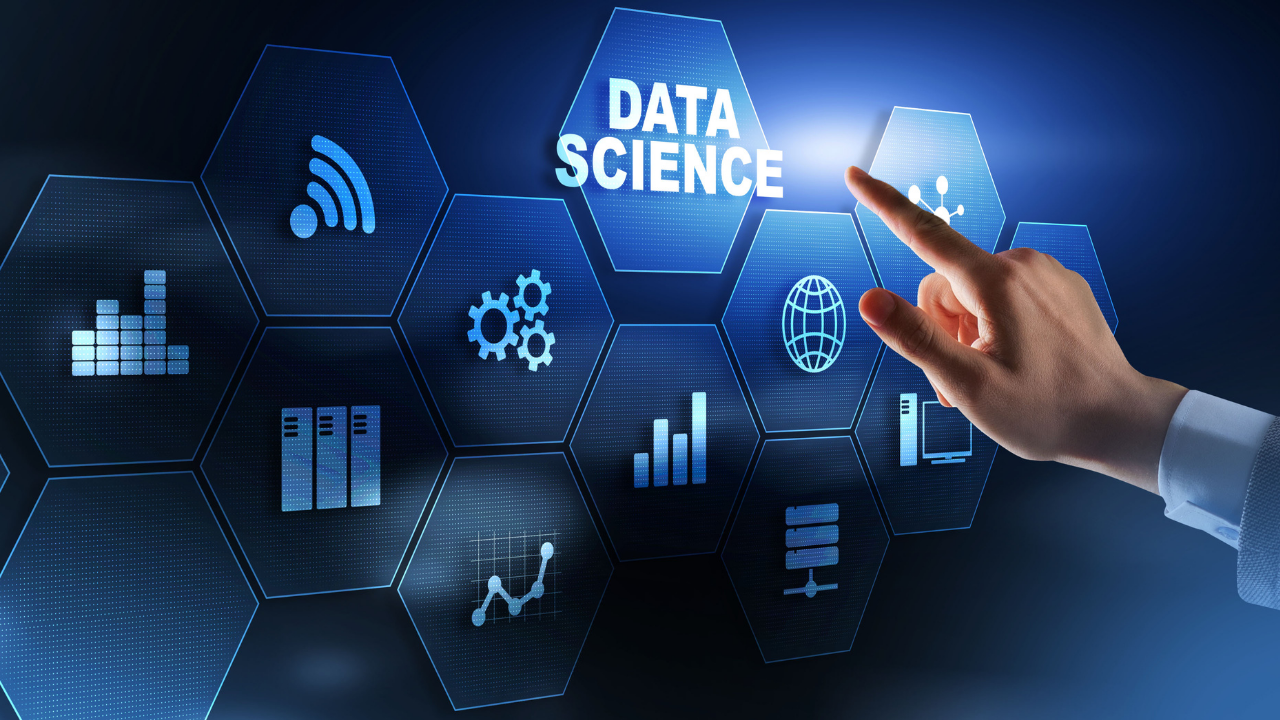
Data is now the most valuable asset for companies in almost every industry. This is because it can predict and analyze a company’s performance and help it succeed. This has been the truth for a decade or so now, and is only becoming more evident over time. Therefore, theories, fields, and educational specializations have also emerged around the world of data. Whether you simply browse TVaninternetdeal.com or surf social media at random, you are generating valuable data for businesses. So, researchers and organizations have come up with new disciplines which handle data in the most incisive ways possible.
Two of these disciplines are data science and data analytics. Many people conflate the two, but they’re actually quite different. These differences go from the surface level to the in-depth methodology, so you should be clear about them. This will help you figure out how to use them for your own purposes or business. So, here’s a basic overview of what the two are, along with their key differences.
What Is Data Analytics?
Data analytics is basically the science where people or systems analyze raw data, and then present a certain set of conclusions. These conclusions take multiple factors in account, and help organizations optimize their processes. In most cases, the techniques of this science have been streamlined and automated into algorithms which do all the legwork and analysis. They then present these conclusions in a decipherable form which is easier for instant understanding. Data analytics comes into play before, during, and after business processes. Many companies invest in dedicated systems and resources for the purpose.
What Is Data Science?
Data science is more of an interdisciplinary field which combines math, statistics, programming, and domain expertise to get insights from data. It extracts this knowledge from structured and unstructured data, and gets answers to various complex business problems. It focuses less on performance, and more on the key issues and concerns of an industry. Its scope is also more relevant to research and development, so it comes into play in the planning stages rather than in the post-process analytical phase.
Also Read: Best Tools for Artificial Intelligence & Machine Learning in 2022
The Differences Between the Two
There are some key differences between data science and data analytics. These may not seem like much, but they actually do matter quite a bit. The two disciplines have very different goals, job descriptions, methodologies, and tools.
Goals
First of all, the key objectives of data analytics and data science are quite different. The former aims to analyze data from ongoing processes, and present conclusions based on it to optimize performance. On the other hand, data science aims to answer the major complexities and concerns involved in various industries. These goals are applicable on a general basis rather than on specific processes.
Job Role
A data analyst and data scientist will have completely different job descriptions. When it comes to a data analyst job, the person will be expected to gauge business trends, clean up data, discover patterns, and develop KPIs and visualizations.
Meanwhile, a data scientist’s work will involve preserving data integrity, exploratory data analysis, using AI and machine learning to gain business insights, and identifying data trends to make future predictions.
Both these job roles are quite different, and cannot be conflate with each other. A data scientist and data analyst will have a completely different skillset, and sometimes may be able to do both functions. However, it is best to keep the two roles separate for good results, especially in larger companies.
Methodology
As the job roles and goals differ significantly, the methodology of these two functions also differs. The former uses existing information to present actionable data. On the other hand, the latter attempts to uncover as new findings and figure out new trends and concerns to address in the future.
Simply put, data analysis is more of a performance overview while data science has a more predictive role. Therefore, their methodology is quite different.
Tools Used
There are also some key differences in tools data scientists and data analysts use. Data analysts use tools like Excel and SQL database, in addition to statistical tools like SAS, Python, or R. Meanwhile, data scientists apply their expertise with tools like Hadoop, Spark, Cassandra, and MongoDB. They also know programming languages such as Scala and Python, and need to have an array of statistical and mathematical tools as well.
So, if you hire data scientists and data analysts, you need to give them the toolkit they need to do their work properly. Investing in these tools will help them get the best possible results and get the most out of data.
In conclusion, there are some major differences between data analytics and data science. Both of these, however, are important for industries and businesses. They help sectors evolve, prosper, and succeed with incisive insights.